An Alternative for Databricks
Which data science challenges do these two machine learning platforms solve?
Databricks vs. Valohai
Machine learning platforms take many forms and usually solve only one or a few parts of the ML problem space.
You could summarize the difference between the platforms as that of a governance platform built for any type of data or technology stack (Valohai) and that of a data analytics platform with a strong focus on Spark (Databricks).
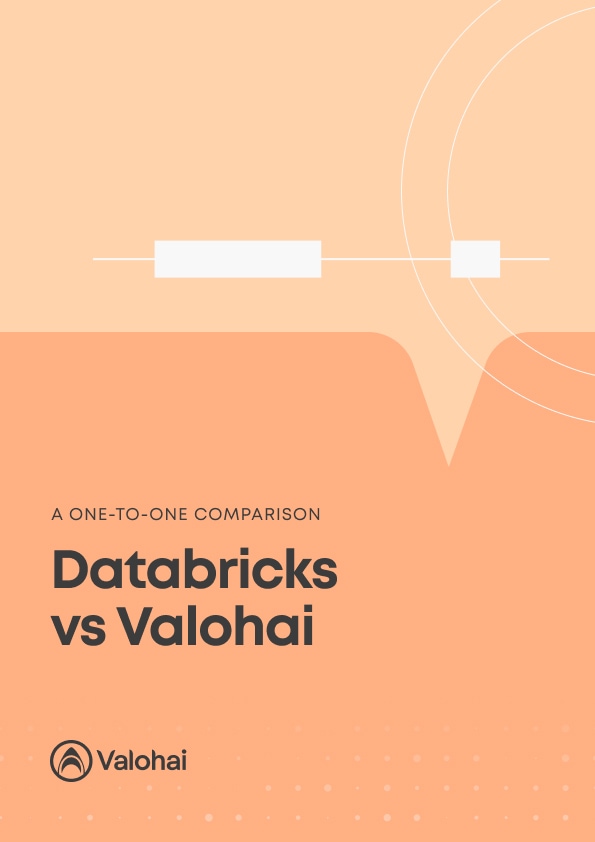
Data management
While Databricks has support for blob storage though its Delta Lakes product, it builds on the Spark query language for accessing that data. Valohai is built for large scale processing of unstructured data with support for frameworks such as Horovod and scales from on-premises to hybrid-cloud data.
Model development
Valohai lets you run your experiments on the exact libraries and environments you need. It also helps you build ML pipeline steps that run in different environments. In Databricks you are bound to certain languages and library versions. Databricks also supports different languages in different notebook cells, which is handy in experimentation but not reproducible for more than one person working on a problem.
Prediction serving
Both Databricks and Valohai ensure role-based access and team management. In terms of governance, Valohai also maintains an up to date audit trail so that you can trace from any experiment, through every script and notebook to the original code and datasets that were used.