Mastering music tracks and podcasts with machine learning
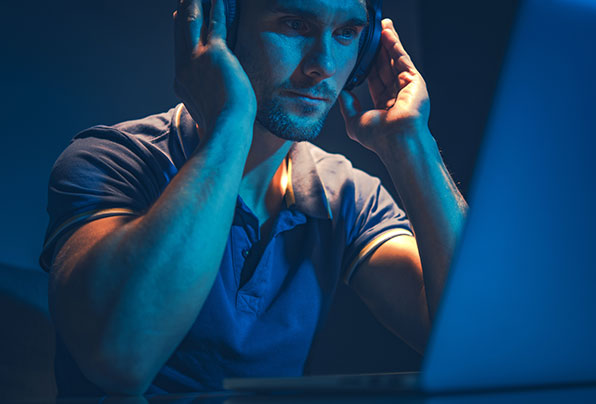
CloudBounce is an exciting SaaS platform using machine learning to intuitively automate the mastering of music tracks and podcasts. With typical software, it usually takes an audio engineer several hours to perfect the sound quality of a track. But with CloudBounce, anyone can upload a composition or even record directly into this innovative solution and get a fully mastered trackback within minutes.
CloudBounce’s current deep learning models are based on a spectrographic representation of sound, and using a raw waveform as neural net input is under research. Their data science team is using a convolutional neural network for supervised classification to label the music style and then predict subsequent mastering choices based on the detected genre.
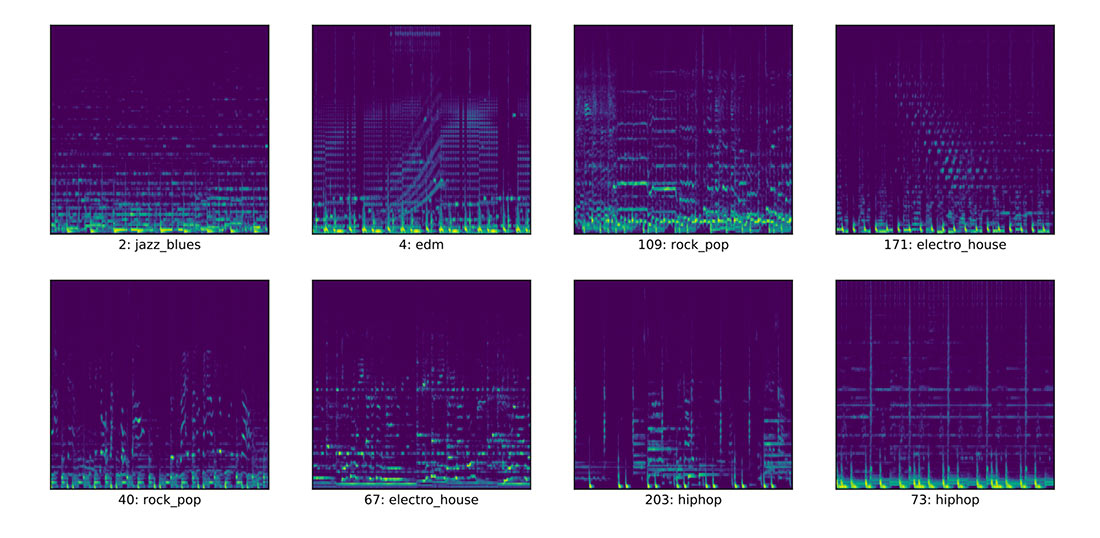
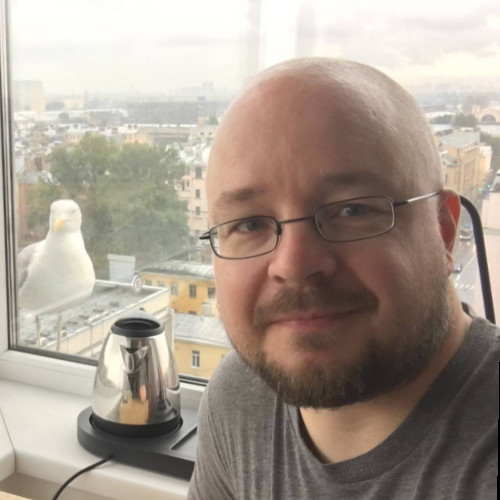
We predict what processing choices a human audio engineer would make and model this mastering process in the neural network. We can use, for instance, adversarial training to learn to mimic human mastering results.
Juho Pennanen – ML Lead, CloudBounceIt’s all about using data scientists time wisely
With a small team like Cloudbounce’s, it’s especially crucial to be efficient in building the ML models, and that’s the main reason why they turned to Valohai. Like with any other machine learning project, this one also requires a vast number of experiments to try different model structures, training parameters, and data preprocessing choices. To find success in any ML project, it’s vital to keep the team’s eye on the ball and concentrated on improving the models without the distraction of building and maintaining the training and inference infrastructure while manually keeping track of the experiments.
We heard good things about Valohai and couldn’t find any other deep learning platform with the same built-in flexibility. I particularly like how the experiments are stored in a reproducible form automatically, including parameters, code, and data selection.
Juho Pennanen – ML Lead, CloudBounceThe road to perfect accuracy
CloudBounce is continuously improving its models’ ability to recognize genres more even more accurately and, as a result, discovering better mastering solutions for its customers. Today, users can adjust the track after CloudBounce’s mastering engine has mastered it. The data science team is planning to use customers’ actions as feedback to decide how well the machine learning model is working and improve the model further based on user actions. This kind of feedback loop can easily be built with Valohai’s pipeline functionality.
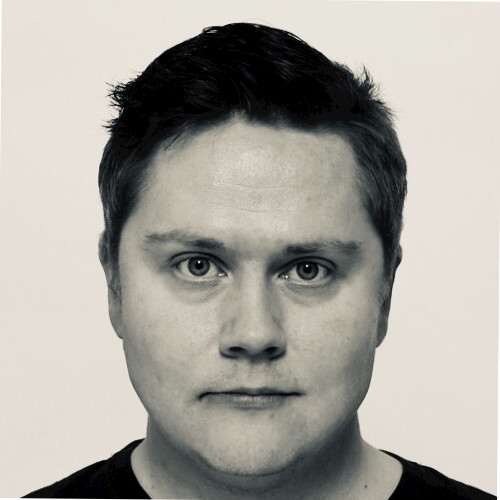
If the user doesn’t edit the track after it has been processed by our mastering engine, it’s a pretty clear sign to us that we’ve succeeded. On the other hand, if everyone adjusts the warmth of a jazz track, for example, it’s a clear indication that we need to train the model again to understand the users better.
Anssi Uimonen – ML Lead, CloudBounceWhile podcast hosts usually have in-depth knowledge about their podcast topic and related content creation, they often are not expert audio engineers themselves. The most important thing to the users is simply the result: to produce a professional-caliber recording with ease, regardless of the technology and mastering options under the hood. CloudBounce eliminates their stress by forgoing the mastering step altogether, enabling users to record straight into the mobile or desktop app and get a high quality mastered track immediately after they finish the session.
Are you a podcaster?
CloudBounce has launched a new product BounceCast for podcasters and content creators BounceCast is the most intuitive way to record and automatically master any podcast or video audio file. The app is available on desktop, and soon on mobile too. To celebrate the launch, they are giving the annual plan at a limited-time, 50% discount.
Grab it here.
Scale your ML development
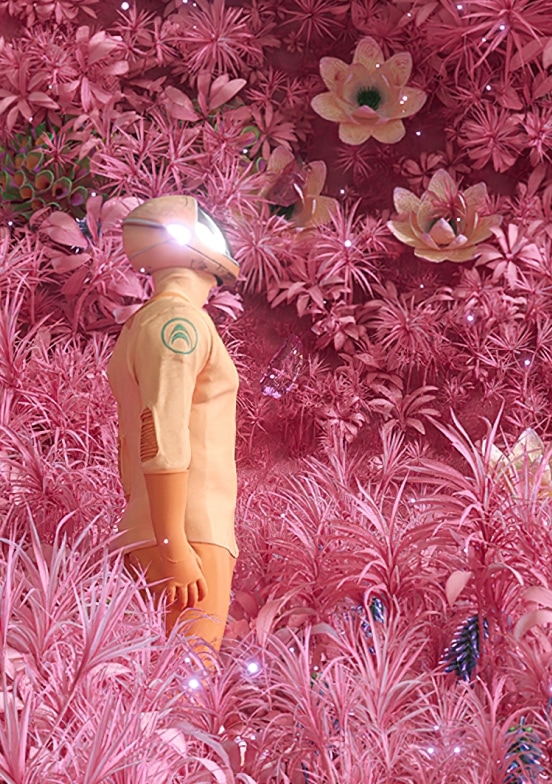
Mistral 7B and YOLOv5
project templatesTake Valohai on a test drive
Valohai is the first and only CI/CD platform for Machine Learning that works seamlessly in hybrid-cloud environments.
Explore how you can shorten your ML development cycles without committing to one cloud provider or compromising on traceability and reproducibility.
Start your trial now