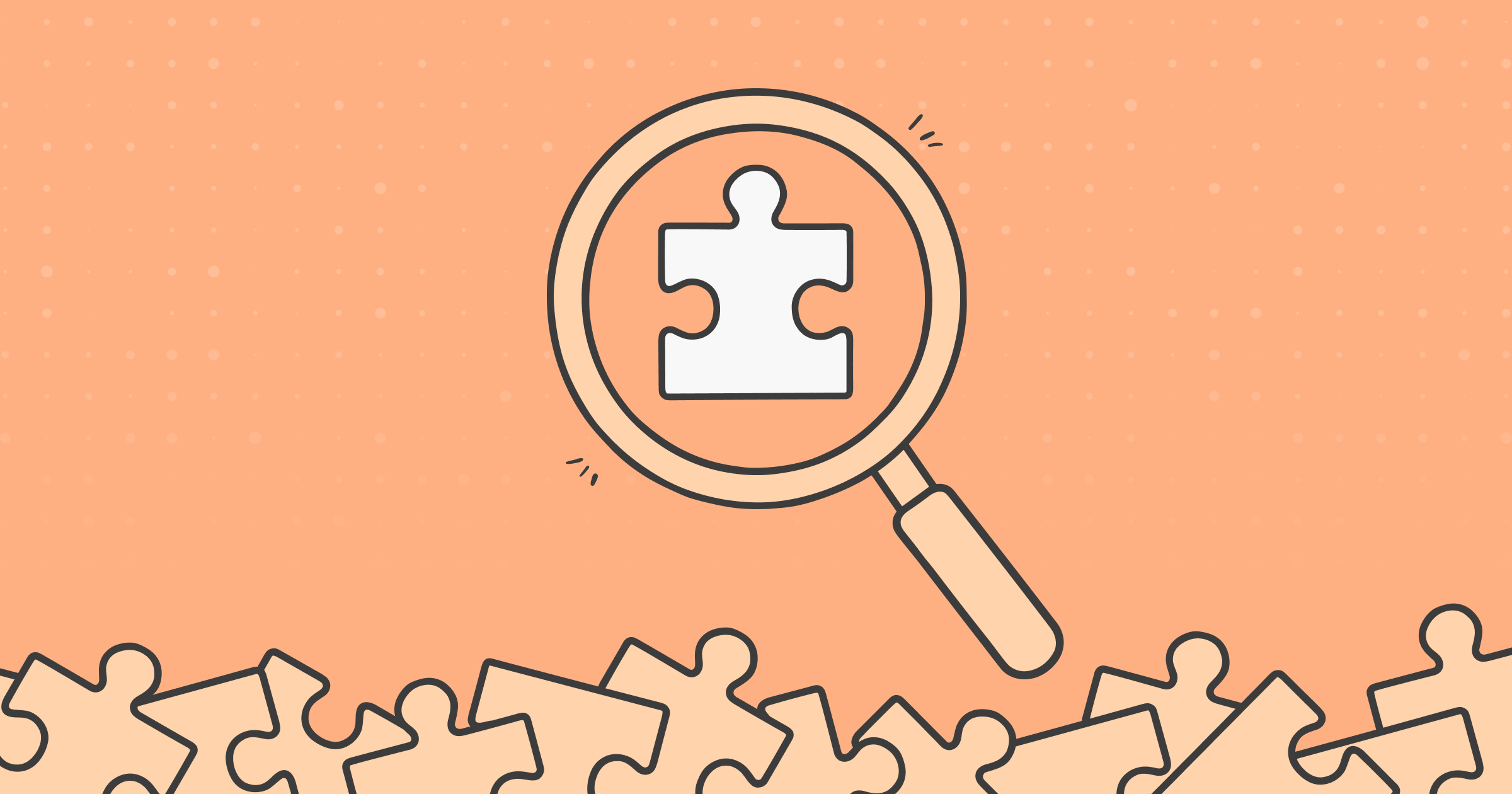
The Hidden Reproducibility Crisis Killing Your ML Team's Productivity (And Your Budget)
by Drazen Dodik | on April 30, 2025Let me tap into your nightmare scenario. You know the one.
Thursday, 4PM. Your team lead messages: "Can you get that object detection model ready for tomorrow's leadership review? The one that hit 96% mAP in your tests last week."
Your stomach drops. You know exactly which model they mean—it was a breakthrough after weeks of tweaking. But where exactly is it?
"No problem," you type back, already feeling the cold sweat forming.
Two hours later, you're staring at results that make no sense. 82% mAP. Then 78%. You've pulled the exact code from your repo. You're using what you think is the same dataset. But something's off.
Midnight finds you crawling through Slack history, hunting for clues. Was it the image augmentation pipeline? Did you forget to log a critical parameter? Maybe it was that CUDA version update from Tuesday?
"I might have tweaked the anchor box sizes locally," you suddenly remember. But did you? And by how much?
Friday morning: bleary-eyed, you present a hastily reconstructed model that barely performs. Your team lead gives you that look—the one that says "I thought you had this handled." Three days of your life vaporized chasing a phantom model that worked perfectly once—but couldn't be resurrected when it mattered.
Sound familiar? You're not alone. This isn't just poor documentation. It's the invisible tax paid by most ML teams: the reproducibility crisis.
The Reproducibility Trap We All Face
Let's be honest—we all know Git isn't enough for ML. While software engineers commit code and call it a day (ok, oversimplification, but let’s go with it), you’re juggling a complexity nightmare.
Your model's performance hinges on that perfect storm of conditions:
- Code (check, Git's got this)
- Data snapshots (which evolve constantly)
- Hyperparameters (spread across config files, CLI flags, and Jupyter cells)
- Random seeds (documented… sometimes)
- Environment dependencies (which someone updated last week)
- Hardware quirks (did that model train on a V100 or A100?)
- Preprocessing configurations (which version of that pipeline again?)
Every ML practitioner has their system—notebooks with markdown cells, experiment trackers, parameter files, containerized environments. Yet somehow, critical details still slip through the cracks when deadlines loom.
We're all fighting the same battle against "ghost experiments"—those runs that performed brilliantly once but vanish into the ether when we need them most. And in computer vision especially, where tiny preprocessing tweaks can dramatically impact results, perfect reproducibility often feels like chasing a mirage.
The Business Impact: Beyond Wasted Compute
The most obvious cost of poor reproducibility is redundant experimentation. But the real business impact cuts deeper:
- Time-to-value delays: When data scientists spend 5+ hours weekly recreating past experiments, that’s a full day of innovation lost.
- Decision quality deterioration: You can’t truly A/B test approaches without reliable experiment replication.
- Compliance nightmares: Try explaining to regulators how your model works when you can’t even reproduce it.
- Onboarding friction: New hires take months instead of weeks to ramp up because institutional knowledge is undocumented.
- Technical debt accumulation: Each unreproducible experiment is a ticking time bomb.
Diamonds are forever, but your colleague who built that model? They’re in Bali. Or at a competitor. Or got hit by the proverbial bus. Either way, you’re left piecing together artifacts from memory.
Most teams don’t realize the full cost until crisis strikes.
Why Well-Intentioned Solutions Still Fail
Most teams patch the problem with tools and “best practices”:
- Notebooks with markdown documentation
- Experiment tracking tools that log metrics
- Parameters files in the repo
- Containerized environments
But these all hinge on human discipline. And under deadline pressure? That discipline crumbles.
You forget to log a change. You run something locally instead of through the official system. You pip install a dependency but never update requirements.txt.
Each small slip renders an experiment irreproducible. And irreproducible experiments might as well not exist—except they still cost time, money, and momentum.
What to Ask Your Team
If your team’s models sometimes feel like one-hit wonders, these questions can surface silent gaps:
When we need to rerun a previous experiment, do we actually know where to start—or do we guess and hope for the best? (If you’ve ever said “just check the Slack history,” this one’s for you.)
Do we have a system that can automatically tell us if an experiment has already been run—and reuse the result? (Or do we rerun things simply because we’re unsure?)
Do we find ourselves running things locally because it’s just easier than using the platform? (If local runs are your default and the platform is your “production step,” it’s a usability issue, not a preference.)
Are we confident that most of our experiments are reproducible—or do we just assume so until we're proven wrong? (If you think it's 80%, it might be closer to 40%.)
What True Reproducibility Looks Like
The solution isn’t better documentation—it’s automation. True reproducibility means:
- Automatic full-context capture: Code, data, environment, parameters, hardware—logged without effort.
- Experiment fingerprinting: Unique signatures to detect true duplicates.
- Intelligent reuse: Re-run prevention by reusing results when possible.
- Environment consistency: Reproducible runtime environments without Docker expertise.
- Cross-infrastructure visibility: Unified tracking across local, cloud, and on-prem systems.
This isn’t about new habits. It’s about infrastructure that handles reproducibility by default.
How Valohai Makes Reproducibility Effortless
Yes, it’s a Valohai blog. But these principles stand, no matter the tool. That said, Valohai happens to nail this. Here’s how:
- Zero-knowledge tracking: Every job—experiment, inference, pipeline—is automatically versioned with full context.
- Complete asset lineage: Every input, parameter, code snapshot, and output logged.
- No-Docker-expertise required: Run in containers, but install packages at runtime however you like—Valohai captures it all.
- Automatic job reuse: Identical jobs are reused to cut compute costs.
- Cross-infrastructure reproducibility: AWS, GCP, Azure, on-prem—everything tracked the same way.
Teams using Valohai cut redundant compute and eliminate the “can we even reproduce this?” stress.
The Real Payoff: More Than Just Saving Money
Yes, reproducibility saves compute (20–30% cost reduction is typical). But the true value is cultural:
- Data scientists iterate fearlessly
- Engineers deploy confidently
- Teams avoid compute queues
- Leaders gain clear visibility
- New hires get productive quickly
Most importantly, it frees everyone from the fear of forgotten experiments. The system remembers, so your team can focus on the future—not reconstructing the past.
The Path Forward: Reproducibility By Default
Reproducibility shouldn't be a goal. It should be a default setting—baked into your ML infrastructure.
With Valohai, it is.
Sounds like a cheesy TV shop ad, doesn't it? "But wait, there's more!"
And yet, here we are. The payoff isn't just budgetary—it's faster delivery, higher quality, and the sweet relief of never again having to explain to your boss why you can't recreate that model from last month.
Your team deserves infrastructure that remembers everything, so they can focus on creating the future instead of reconstructing the past.
Let's Talk
If you're wondering how much irreproducible work is hiding in your ML workflows—and what it's really costing you—let's talk.
Want to see how Valohai ensures perfect reproducibility for every ML workload while speeding up your path to production? Connect with me on LinkedIn or book a time.