MLOps Basics
What is MLOps?
MLOps (machine learning operations), sometimes also written as ML Ops, is a practice that aims to make developing and maintaining production machine learning seamless and efficient. While MLOps is relatively nascent, the data science community generally agrees that it’s an umbrella term for best practices and guiding principles around machine learning – not a single technical solution.
Why use MLOps?
The goal of MLOps is to reduce technical friction to get the model from an idea into production in the shortest possible time to market with as little risk as possible.
Traditionally, machine learning has been approached from a perspective of individual scientific experiments predominantly carried out in isolation by data scientists. However, as machine learning models become part of real-world solutions and critical to business, we will have to shift our perspective, not to depreciate scientific principles but to make them more easily accessible, reproducible, collaborative, and most importantly to increase the speed at which machine learning capabilities can be released.
The reality is that only a model running in production can bring value. Models have zero ROI until they can be used. Therefore, time to market should be the number one metric to look at and optimize for any commercial ML project.
Priorities in ML for the next 3 months (as of May 2020)Source: State of ML 2020, 330 respondents
In May 2020, we surveyed 330 data scientists, machine learning engineers, and managers in a broad range of companies to ask what they were focused on for the next three months and what major obstacles they were faced with. Half of the respondents said that they were focused on developing models for production use, and over 40% said they would be deploying models to production.
Automating retraining of models and monitoring models in production were still not relevant for most respondents, which speaks to the practice of MLOps of being still relatively nascent. Production models raise new challenges, not just for data scientists but for the extended team of engineers, product managers, and compliance officers, which will need to be solved collaboratively.
In most real-world applications, the underlying data changes constantly, and thus models need to be retrained, or even whole pipelines need to be rebuilt to tackle feature drift. Business and regulatory requirements can also change rapidly, requiring a more frequent release cycle. This is where MLOps comes in to combine operational know-how with machine learning and data science knowledge.
MLOps principles and best practices
MLOps encourages collaboration
When approached from the perspective of a model – or even ML code, machine learning cannot be developed truly collaboratively as most of what makes the model is hidden. MLOps encourages teams to make everything that goes into producing a machine learning model visible – from data extraction to model deployment and monitoring. Turning tacit knowledge into code makes machine learning collaborative.
MLOps encourages reproducibility
Data scientists should be able to audit and reproduce every production model. In software development, version control for code is standard, but machine learning requires more than that. Most importantly, it means versioning data as well as parameters and metadata. Storing all model training related artifacts ensures that models can always be reproduced.
MLOps encourages continuity
A machine learning model is temporary. The lifecycle of a trained model depends entirely on the use-case and how dynamic the underlying data is. Building a fully automatic, self-healing system may have diminishing returns based on your use-case, but machine learning should be thought of as a continuous process and as such, retraining a model should be as close to effortless as possible.
MLOps encourages testing and monitoring
Testing and monitoring are part of engineering practices, and machine learning should be no different. In the machine learning context, the meaning of performance is not only focused on technical performance (such as latency) but, more importantly, predictive performance. MLOps best practices encourage making expected behavior visible and to set standards that models should adhere to, rather than rely on a gut feeling.
Do you think something is missing from the above, email us with your opinion.
How to implement MLOps principles into your project?
1. Recognize the stakeholders.
The size and scope of real-world machine learning projects have surely surprised most – if not all of us. What seems like a straightforward task of gathering some data, training a model and then using it for profit ends up becoming a deep rabbit hole that spans from business and operations to IT. A single project covers data storage, data security, access control, resource management, high availability, integrations to existing business applications, testing, retraining, etc. Many machine learning projects end up being some of the biggest multidisciplinary and cross-organizational development efforts that the companies have ever faced.
To properly implement an MLOps process, you’ll have to recognize the key people and roles in your organization. We’ve talked to countless organizations in the past four years, and while each case is unique, there tends to be a combination of these roles that contribute machine learning projects:
- Data scientists (duh!)
- Data engineers
- Machine learning engineers
- DevOps engineers
- IT
- Business owners
- Managers
These roles are not necessarily one per person, but rather a single person can - and in smaller organizations often has to - cover multiple roles. However, it paints a picture of who you’ll need to identify to gather your specific requirements and use your organization’s resources. For example, you must talk to business owners to understand the regulatory requirements and IT to grant access and provision cloud machines.
2. Collaboratively agree on your MLOps process.
A few sound principles that the whole team agrees on are a great starting point for implementing MLOps. For example, the four principles we suggested above may all be obvious to you, but they may not be for your colleague or your future colleagues.
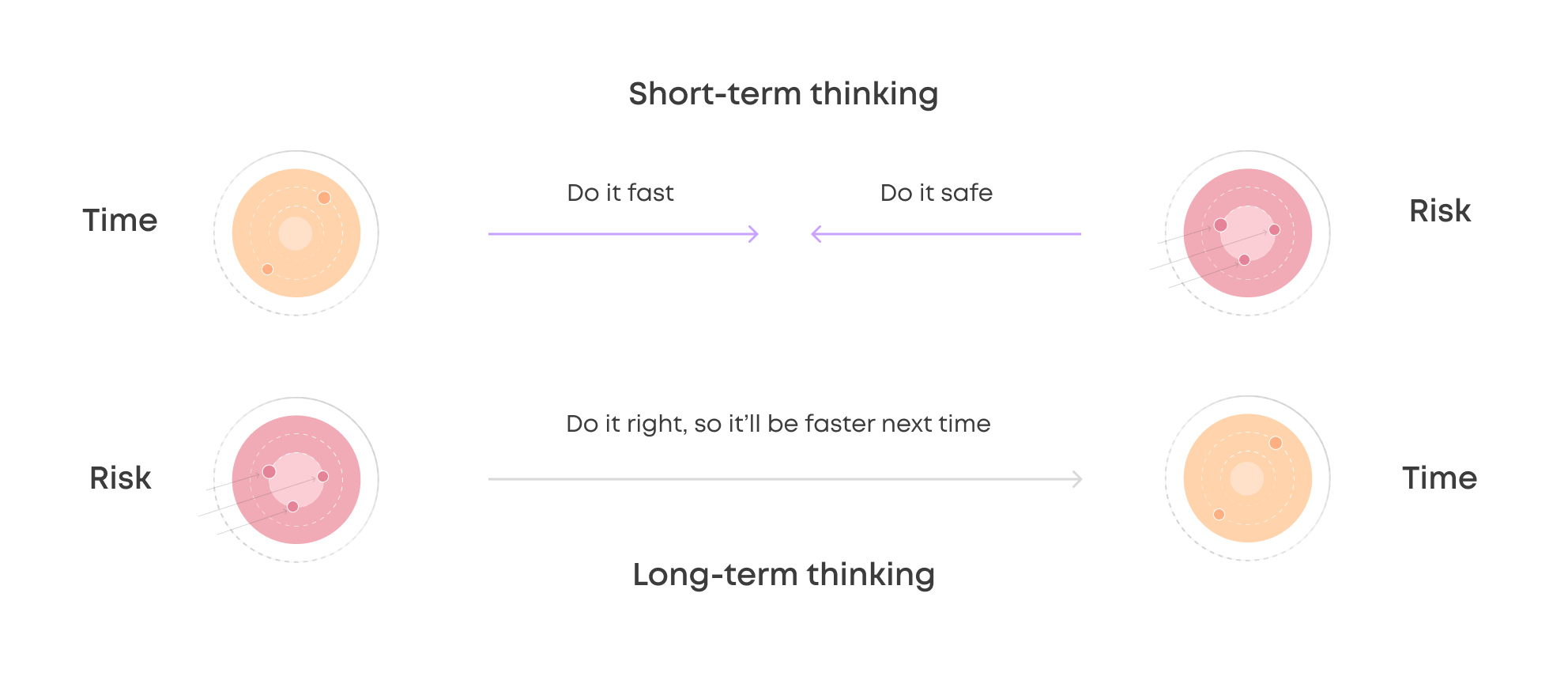
It's too early for MLOps is a common argument we hear.
We hear the argument that it’s too early to think about MLOps frequently. Still, in most of these cases, the organization is already making significant investments into machine learning and sees ML as a core part of their future. Short-term thinking may encourage data scientists to work in an ad-hoc manner, but if a few decades of software engineering has taught us anything, it’s that it’ll bite you in the behind later on. In the long-term, doing it right will lead to time saved as work doesn’t have to be done and done again.
While most people get excited about tools and technologies, it’s important to first agree on why you’d want to implement them. This is especially true if you need to convince others to use the same tools.
3. Adopt tools and infrastructure to implement the MLOps process.
MLOps tools are plentiful. A recent article listed over 300 tools in this space (most of them have names that sound like Pokémon), but if you’ve agreed with your team how you will work, finding the correct tooling will be so much easier.
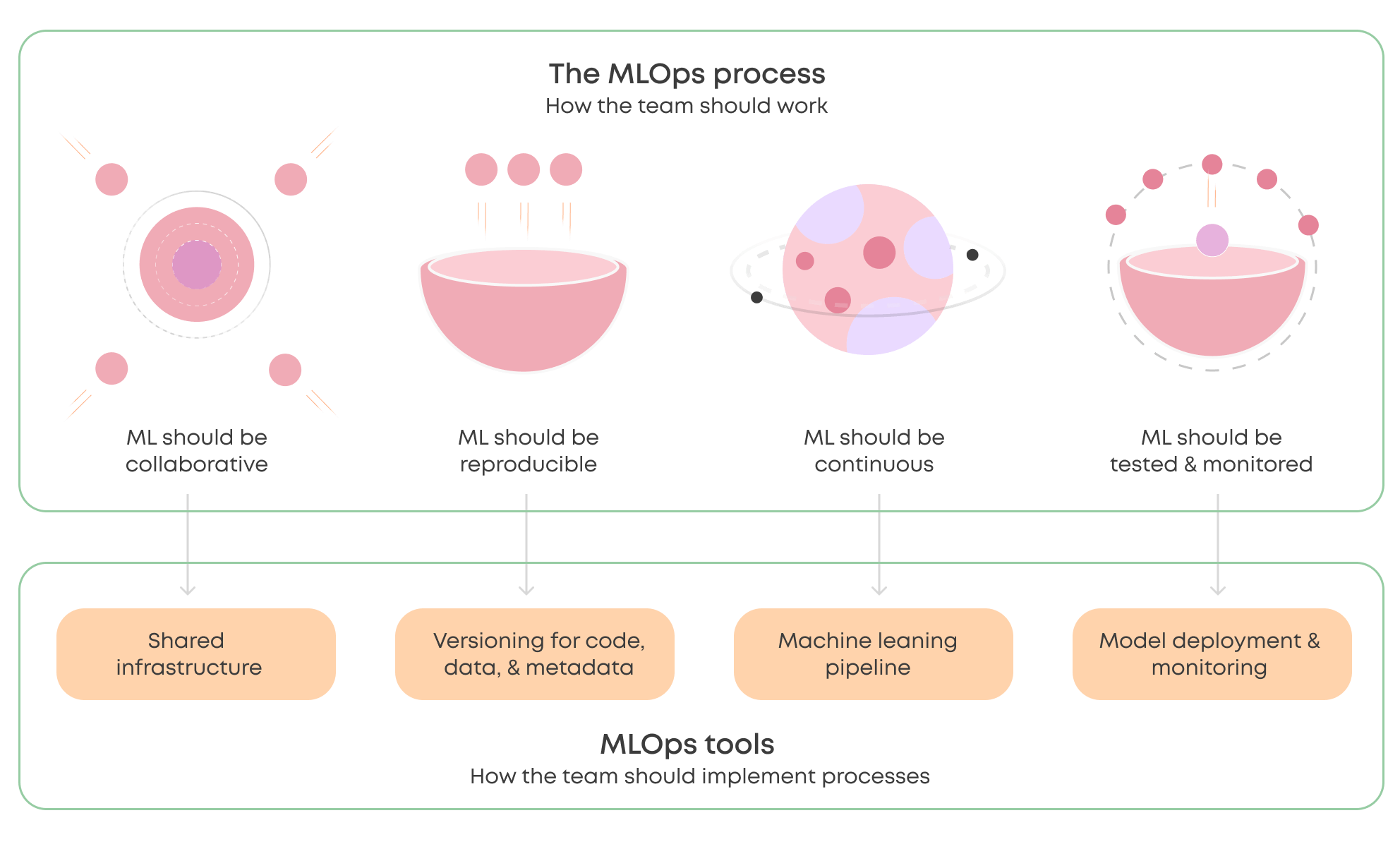
Your process and requirements should lead your tooling selection.
Here are a few ideas on how to implement our four principles practically with MLOps tooling. Your solutions may differ as these too are very contextual.
- MLOps encourages collaboration: If your teammates work on their local environments, shared infrastructure will get them out of their sandboxes.
- MLOps encourages reproducibility: If your teammates have unique ways to document (or not document 😱) their work, a tool that tracks experiments automatically should unify that easily.
- MLOps encourages continuity: If your models are trained through ad-hoc workflows, a machine learning pipeline should make it easier to retrain them. Data drift always hits when you least expect it.
- MLOps encourages testing and monitoring: If you don’t know how well your models perform in the real world, consider implementing monitoring and closing the loop with automatic retraining.
As said, there are plenty of proprietary and open-source products that solve parts of the machine learning lifecycle. When comparing platforms in the MLOps space, you’ll often run into apples to oranges comparisons. For example, comparing KubeFlow and Valohai is tricky because the former is an extendable, open-source solution requiring weeks to adopt, and the latter is a managed, proprietary solution.
There are many approaches to ML infrastructure that can work, whether it’s coupling specialized systems or using a single multipurpose platform. However, infrastructure work should start as early as possible to avoid situations where your team has models in production, but how the models were produced isn’t well documented and each new release is becoming increasingly difficult to do.
To dig in deeper into how to choose your tooling, we’ve written two guides: Build vs. Buy and MLOps Platform Comparison. Additionally, we recommend using the MLOps Stack template to map out your tool selections.
4. Automate, automate, automate.
When moving from POC to production, there is a significant change in mindset you’ll have to make. The ML model is no longer the product; the pipeline is.
While machine learning projects often start with one huge notebook and some local data, it’s essential to begin splitting the problem solving into more manageable components; components that can be tied together but tested and developed separately.
This ability to split the problem solving into reproducible, predefined and executable components forces the team to adhere to a joined process. A joined process, in turn, creates a well-defined language between the data scientists and the engineers and also eventually leads to an automated setup that is ML equivalent of continuous integration (CI) – a product capable of auto-updating itself.
An end-to-end, automated machine learning pipeline ensures that every change – in either data or code – is (or can be) deployed to production without it turning into a special project.
Summary
As mentioned before, MLOps is not dependent on a single technology or platform. However, technologies play a significant role in practical implementations of MLOps, similarly to how adopting Scrum often culminates in setting up and onboarding the whole team to e.g. JIRA. Therefore, the project to rethink machine learning from an operational perspective is often about adopting the guiding principles and making decisions on infrastructure that will support the organization going forward.
The result of MLOps should be a supercharged release cycle of machine learning capabilities. It’s paramount to understand that MLOps is a combination of people and technologies; people have to be willing to automate and document their work and tools have to make it easy.
For more MLOps advice, read our guide on how to choose the right MLOps platform or download our Practical MLOps eBook.
The best of the best
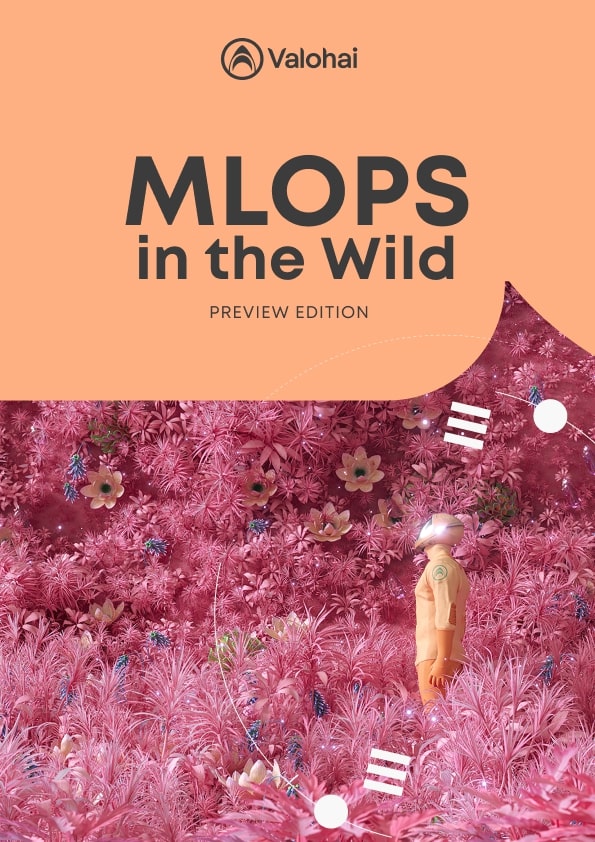
MLOps in the Wild
A collection of MLOps case studiesSkimmable. Inspirational.
The MLOps space is still in its infancy and how solutions are applied varies case by case. We felt that we could help by providing examples of how companies are working with tooling to propel their machine learning capabilities.
Think of this as a lookbook for machine learning systems. You might find something that clicks and opens up exciting new avenues to organize your work – or even build entirely new types of products.
Download