Choosing the Best MLOps Platform: a Comprehensive Comparison of MLOps Platforms
Congratulations, you’ve decided to invest in MLOps. You might be in a situation where you already have machine learning models in production, or you know you’ll go to production soon. Either way, you know machine learning will be key for your success in the future, and anything that’ll accelerate your speed to market is worth investing in. In other words, you are looking for an MLOps platform.
What is an MLOps platform?
The definition of MLOps is fuzzy and the definition of an MLOps platform is even fuzzier. The field is still relatively nascent and so are the tools. We expect to see the developer tooling space for machine learning to go through several iterations before a more commonly understood categories are formed, like what has happened in the DevOps space.
In this article we’ll go through some of the more popular platforms under the MLOps umbrella, with the criteria that the MLOps tool should offer functionality in one of the following areas:
- Tracking and versioning
- Machine learning pipelines
- Model deployment
The platforms we’ve chosen for our analysis are ClearML, cnvrg.io, Dataiku, Datarobot, Iguazio, Sagemaker, Seldon and Valohai from the managed side, and Flyte, Kubeflow, MLflow and Metaflow from the open-source side. This is by no means an exhaustive list of all the MLOps tools out there.
Most of these are tools that describe themselves as end-to-end MLOps platforms and cover the three areas we outlined. End-to-end MLOps platforms, like Valohai, bring most of the model lifecycle under a single workflow, while MLflow, Flyte, Metaflow and Seldon focus on a single area of the lifecycle.
How to compare MLOps products?
Comparing machine learning and MLOps platforms is incredibly tricky as these products are complex. Generally, the differences between platforms can only be fully realized with real-world testing with an actual use-case. The marketing messaging for these platforms is very similar so getting clear differentiation is difficult (note to self, think different 😉).
One way to compare MLOps platforms is to compare features. Many of these platforms are quite identical in top-line features, but how the features work in practice vary wildly. Therefore one might compare the platforms by how they position themselves.
Another way would be to look into whether you need to buy a ready-made MLOps platform or build something from scratch. The right answer depends on your team and your use case. Most technical decision-makers tend to skew towards building because it is their bread and butter, but we want to encourage critical thinking around the subject.
Thus, In this article, we compare popular managed and open-source MLOps platforms by their focus and their fit as a build or buy MLOps solution.
MLOps platforms compared by focus
Traditional machine learning focus vs. deep learning focus
Products that focus on traditional machine learning are built for structured data (SQL, Excel, etc.) and efficient processing through, for example, Spark. These MLOps platforms may also offer additional capabilities for data analysis and data manipulation in visual tools.
Try Valohai free for 14 days and see how seamless the workflow from experimentation to production can be.
Free TrialOn the other hand, MLOps platforms for deep learning are built to handle massive amounts of unstructured data such as images, videos, or audio. Significant emphasis is placed on utilizing powerful GPU machines as these models may take days to train even on the most powerful hardware.
On the traditional machine learning side, we have products like Metaflowwhich is ideal for tabular data while Valohai sits on the deep learning side with a heavy focus on machine orchestration.
Exploration focus vs. productization focus
Generally, MLOps, as a concept, is focused on machine learning production.
Exploration focused platforms emphasize data analytics, experiment tracking, and working in notebooks, while productization focused platforms primarily concentrate on machine learning pipelines, automation, and model deployment.
Seldon, Flyte, and Metaflow are most strictly production focused. Flyte and Metaflow focus on building production pipelines while Seldon is for model versioning and model deployment only, not training models. MLFlow, on the other hand, is more focused on experiment tracking, and Dataiku has a lot to offer on the data analysis side of things.
Citizen data scientist focus vs. expert data scientist
Citizen data scientists are more subject matter experts rather than technical experts. Some MLOps platforms are focused on this category of users as they offer capabilities for teams with less engineering expertise to build and deploy machine learning models. These platforms focus on visual tools and access through a Web UI.
On the other end, we have platforms focused on expert data scientists with engineering expertise or teams that contain significant data science and engineering expertise. These platforms tend to avoid proprietary code as much as possible and focus on supporting as many existing languages and frameworks as possible. Web UI may not be as big of a focus as expert users tend to opt for command-line interface (CLI) or API when integrating the platform with existing tools.
Datarobot roots for the citizen data scientist with its heavy emphasis on AutoML, while open-source platforms like Flyte, Metaflow, and Kubeflow are more suited for large teams of expert data scientists with deep engineering/DevOps skills. Most managed platforms fall somewhere between without requiring the same DevOps as the open-source platforms. Valohai and cnvrg.io, however, put a heavier emphasis on remaining technology agnostic and interoperable.
Specialized approach vs. end-to-end approach
Most of the MLOps platforms listed in this article approach MLOps from an end-to-end perspective, meaning the user should be able to automatically train, evaluate, and deploy a model in a single platform. For a real apples to apples comparison, those with a specialized approach should be excluded, but we included a few that often come up in MLOps platform evaluations.
There is a place for specialized products, but you’ll generally need to complement them with other products to form an end-to-end MLOps platform.
Seldon, Flyte, and Metaflow standout in this comparison as they are more narrowly focused in either pipelines or deployment. Datarobot is not genuinely end-to-end except in AutoML use cases, and MLFlow is only starting to move to an end-to-end approach.
MLOps platforms summary overview
Name | Category | Description | Areas | Focus |
---|---|---|---|---|
AWS Sagemaker | Managed | Build, train, and deploy machine learning (ML) models for any use case with fully managed infrastructure, tools, and workflows | Tracking and versioning Pipeline orchestration Model deployment | AWS |
ClearML | Managed, Open-source | MLOps with only 2-lines-of-code. Easily Develop, Orchestrate, and Automate ML Workflows at Scale. | Tracking and versioning Pipeline orchestration Model deployment | Experimentation, Structured data |
cnvrg.io | Managed, Open-source | An end-to-end machine learning platform to build and deploy AI models at scale | Tracking and versioning Pipeline orchestration Model deployment | Technology agnostic |
Dataiku | Managed | Dataiku is the platform democratizing access to data and enabling enterprises to build their own path to AI in a human-centric way. | Tracking and versioning Pipeline orchestration Model deployment | Enterprise, Data Analysis, Business Intelligence |
Datarobot | Managed | DataRobot is the leading end-to-end enterprise AI platform that automates and accelerates every step of your path from data to value. | Tracking and versioning Pipeline orchestration Model deployment | AutoML, Enterprise |
Iguazio | Managed, Open-source | The Iguazio Data Science Platform automates MLOps with end-to-end machine learning pipelines, transforming AI projects into real-world business outcomes. | Tracking and versioning Pipeline orchestration Model deployment | Structured data |
Seldon | Managed, Open-source | Deploy machine learning models at scale with more accuracy. 85% Faster. | Model deployment | Enterprise, Deployment |
Valohai | Managed | Train, Evaluate, Deploy, Repeat. Valohai is the MLOps platform that can automate everything from data extraction to model deployment. | Tracking and versioning Pipeline orchestration Model deployment | Deep Learning, API-first, Technology agnostic |
Flyte | Open-source | Lyft’s Cloud Native Machine Learning and Data Processing Platform, Now Open Sourced | Pipeline orchestration | Pipelines |
Kubeflow | Open-source | The Kubeflow project is dedicated to making deployments of machine learning (ML) workflows on Kubernetes simple, portable, and scalable. | Tracking and versioning Pipeline orchestration Model deployment | Community, Extendability |
Metaflow | Open-source | A framework for real-life data science | Tracking and versioning Pipeline orchestration | Pipelines |
MLFlow | Open-source | MLflow is an open-source platform for managing the end-to-end machine learning lifecycle. | Tracking and versioning | Experimentation, Spark |
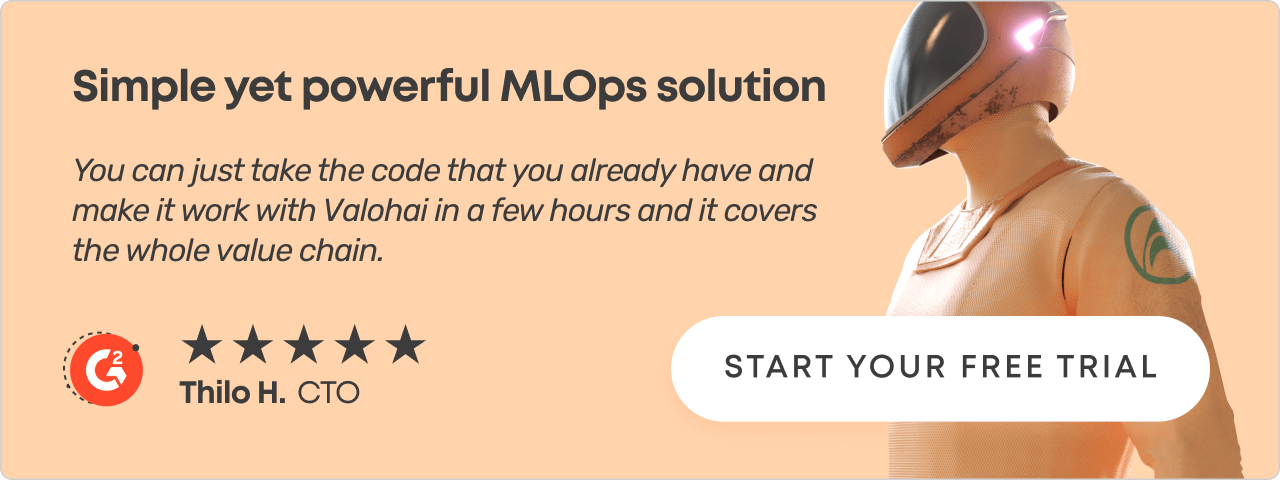
MLOps platforms summary overview
Another discussion that relates to MLOps platforms is whether to build or buy a platform, and this don’t really represent two different options but countless different permutations, e.g.:
- Buy a single, end-to-end managed MLOps platform
- Buy tools for some areas, adopt open-source for others
- Adopt a single, end-to-end open-source MLOps platform
- Build something from scratch (not something we’d recommend)
On our list we have 7 end-to-end, managed MLOps tools which cover at least a large part of the model lifecycle. This would be one way to buy MLOps capabilities. You could also consider buying a point-solution for a certain area (like model deployment) which you consider most difficult to implement and complement this with existing or open-source tools.
Another route may be that you are already using for example MLflow for experiment tracking and model versioning, and you want to complement it with orchestration and model deployment. We see this path quite often with Valohai users.
Although we encourage teams that are seriously investing into machine learning to look at end-to-end MLOps solutions such as Valohai, but whether build or buy is the right choice comes down to what tools best fit your specific needs and what are your most limited resources. You may want to look at our article on the pros and cons of the managed and open-source platforms.
Which MLOps platform works best for your use-case?
TL; DR: The Best MLOps Platform
The right MLOps platform, in the end, comes down to your specific use case and also your particular strengths. If you evaluate MLOps platforms, you may want to consider the scales presented above and figure out which end you lean on each. You should also figure out if there is an essential scale for you, which we didn’t consider.
There are 1001 different ways of making comparisons. We suggest you focus on the functionality you need and financial validity of buying or building an MLOps platform that offers such functionality.
We’ve made a bunch of more specific comparisons between the popular MLOps platforms:
Valohai comparisons
- Valohai vs. Kubeflow - Comparison Whitepaper
- Valohai vs. SageMaker - Comparison Whitepaper
- Valohai vs. Domino Data Lab - Comparison Whitepaper
Kubeflow comparisons
- Kubeflow vs. MLflow - Comparison Article
- Kubeflow vs. Metaflow - Comparison Article
- Kubeflow vs. Sagemaker - Comparison Article
Bear in mind, all of these platforms are continually evolving in features and market positioning. For any feedback, don’t hesitate to email us at hello@valohai.com.
For more information about the Valohai platform, see our product page.
The best of the best
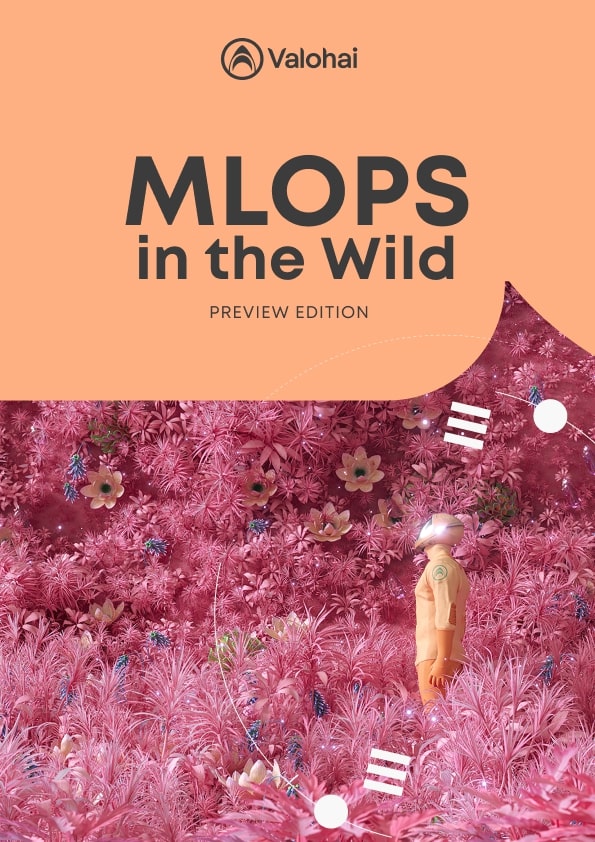
MLOps in the Wild
A collection of MLOps case studiesSkimmable. Inspirational.
The MLOps space is still in its infancy and how solutions are applied varies case by case. We felt that we could help by providing examples of how companies are working with tooling to propel their machine learning capabilities.
Think of this as a lookbook for machine learning systems. You might find something that clicks and opens up exciting new avenues to organize your work – or even build entirely new types of products.
Download