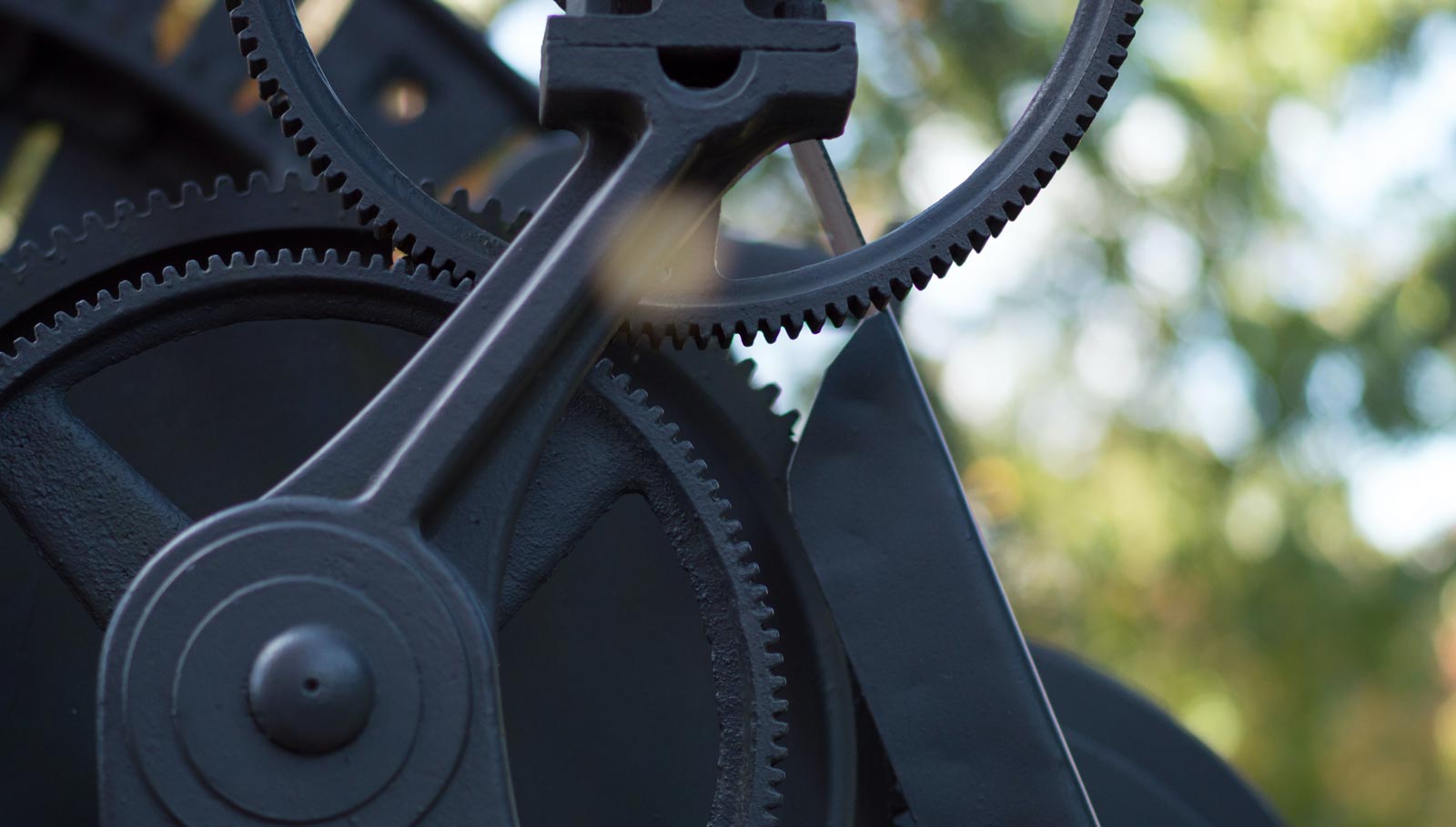
If you are involved with production machine learning in any way, understanding MLOps is essential. For those who are new to the topic, we highly recommend to read our guide on MLOps basics first. For people with some software development experience, the easiest way to understand MLOps is to compare it to DevOps. This guide will help you understand both terms, as well as how they are similar and different.
DevOps and MLOps aim to bridge the gap between development and operations
DevOps, development operations, refers to bringing together the development, testing, and operational aspects of software development. MLOps, or machine learning operations, took many principles from DevOps, which was developed first, and referres to the methods used to streamline the machine learning life cycle from beginning to end.
MLOps builds on the concepts of DevOps and adds missing pieces. While the concepts of MLOps are still being developed, we can already see similar foundational concepts being put into practice as in DevOps. A good example of this is data versioning. In ML, it's simply not enough to versioning the code that trains the model but also the data used. While this is understood, the practical applications of data versioning are still very varied (unlike code versioning, i.e. Git).
In a nutshell, both DevOps and MLOps encourage and facilitate collaboration between people who develop (software engineers and data scientists), people who manage infrastructure, and other stakeholders. Both emphasize process automation in continuous development so that speed and efficiency are maximized.
DevOps and MLOps Comparison
Now that you understand the key concepts and underlying principles of both approaches, let's take a closer look at similarities and differences between MLOps and DevOps.
Similarities between MLOps and DevOps
Firstly, both DevOps and MLOps are about streamlining processes. DevOps brings together the development, testing, and operational aspects of software development. It aims to turn these siloed processes into a continuous set of cohesive steps within an organization. In a similar way, MLOps are the methods used to streamline the machine learning life cycle from beginning to end. It aims to bridge the gap between design, model development, and operations to shorten turnaroun times in ML development.
Secondly, DevOps and MLOps are about communication. For DevOps clear communication is crucial for automation of processes, continuous delivery, and feedback loops since it relies on smooth cooperation between departments and a set of tools that solidify and facilitate these processes in a visible way (for example, CI/CD systems). And for MLOps communication is a base for collaboration between system administrators, the data science teams, and other departments throughout the organization bringing a common understanding of how production models are developed and maintained.
Differences Between DevOps and MLOps
Although they have some similarities, it is impossible to take DevOps tools and use them to operationalize machine learning. Some requirements are specific to machine learning.
Versioning for Machine Learning
With DevOps, code version control is utilized to ensure clear documentation regarding any changes or adjustments made to the software being developed. With machine learning, however, the code isn't the only changing input. Data is the other critical input that'll need to be managed, as will parameters, metadata, logs, and finally, the model.
Hardware Required
Training machine learning models, especially true for deep learning, tend to be very compute-intensive. For most software projects, the build time is entirely irrelevant, and thus the hardware on which it's done is also irrelevant. Larger models, however, can take anywhere from hours to weeks to train, even on most GPU machines cloud vendors offer, meaning that an MLOps setup needs to be much more sophisticated in what kind of machines it can manage.
Continuous Monitoring
Monitoring is a part of good DevOps practices as well. In the past few years, site reliability engineering (SRE) has been all the rage highlighting the importance of monitoring in software development. The difference between monitoring in DevOps and MLOps is that software doesn't degrade, while machine learning models do.
Once a model is deployed into production, it begins to generate predictions from new data that it receives from the real world. This data will continue to change and adapt as the business environment does, resulting in model degradation. MLOps provides for procedures that facilitate continuous monitoring and retraining so that the algorithms may continue to be used in production.
MLOps: Building on DevOps
Today there is simply no successful software company that can operate without adopting any DevOps principles and tooling. Similarly, going forward, there will be simply no way to manage the development and productization of machine learning models without some shared MLOps principles and tooling. Like DevOps today, there'll be different flavors of MLOps, but a systematic approach is simply a must.
MLOps concepts will likely be built on top of the DevOps stack.
We'll have to wait and see how the MLOps landscape will differ from the DevOps landscape but it's clear that there is a connection between the two. It's even possible that as machine learning becomes a standard part of many software products, DevOps and MLOps merge and things like data versioning and continuous training pipelines just get added to existing technology stacks.