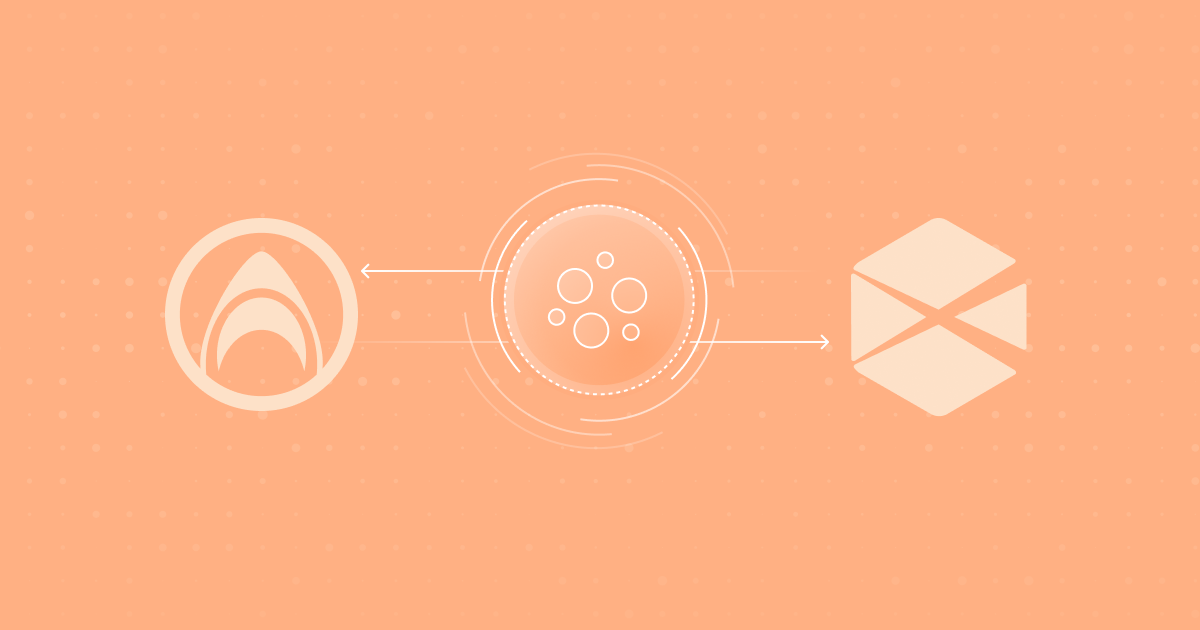
In 2022, Valohai released many new features. That's why our team held a webinar in March 2023 to go through the releases of 2022, sharing some tips and tricks on how to stay up-to-date with the newest features. The highlight of the event was a presentation by Andres Hernandez, Principal Data Scientist at KONUX, who spoke about his experience with Valohai datasets.
Andres Hernandez, Principal Data Scientist at KONUX, speaking about Valohai datasets
In this article, we have summed up the key takeaways from his presentation to help all Valohai users and those who are only considering using the platform to get on board with the datasets and see how KONUX streamlines their operations utilizing this feature.
But first things first.
Meet KONUX
KONUX combines Industrial IoT (IIoT) and machine learning to transform railway operations. Their platform allows railway operators to monitor their rail infrastructure and inform them about issues before they happen. The company's technology is utilized by some of the largest railway companies in the world, including the railways in Germany and the United Kingdom.
Their IIoT devices mounted on the rail bearers measure vibration from passing trains. In addition, KONUX has built intelligent analytics that can detect and identify trains and railcars on the rail from the sensor data and alert to any abnormalities. This helps the railway operators dispatch maintenance crews to ensure safety, improve availability and prolong the lifespan of the existing infrastructure.
You can learn more about them from their website or our dedicated case study. And we will now take a closer look at how the KONUX team organizes their work and what challenges there are.
Getting on track fast with traceability
There are 15 data scientists who are working on four to six different projects in parallel. Each of these projects may last anywhere between three months to a year, sometimes with long pauses in them. This means that oftentimes people who join any given project have either lost track of what has been done since they left or they are entirely new to the project and know nothing about it. However, one needs to know, for example, what was the latest model for a particular client. Answers to such questions should be fast to find within minutes without having to ask around.
The same goes for personal projects. At KONUX, team members are encouraged to dedicate about 15% of their time to independent tasks. Thus, when you get back to your side project, you should be able to easily access information about what has been done so far. Otherwise, the work is just not efficient.
So, first of all, we are looking at the traceability problem here. And, according to Andres, the solution to it consists of two parts: keeping proper documentation and using common tools.
Ways to ensure traceability as presented by Andres Hernandez
Keeping clear documentation
Documentation should always be in order. And datasets should be part of your documentation. If you are to work on a project, you should not waste time going around and asking people questions about it. You should be able to see in the documentation what is the latest dataset used to train a model. Or, for example, what is the execution that we used in production for a specific client? Executions should be tagged accordingly, and so on.
Using common tools
Since people in KONUX tend to work independently for long periods of time, it is crucial that they do not reinvent the wheel every time they get back to a specific project. Thus, it is great to have a central library.
Moreover, the team also relies on programmatic dataset creation. Usually, there are two types of tasks at KONUX. One is producing labels. And the other one is producing the model. The idea is that all the team members know that there are two pipelines, and that the last part of each of them programmatically creates a new dataset version, and those datasets are easy to find in the UI or using the latest-tag.
With Valohai, it is super easy to make this magic happen. It is possible to have a rule where labels produced by the pipeline are grouped into a new dataset version. And everyone knows that this is THE dataset to use further on because their jobs can always point to "latest" version.
"The biggest benefit of using Valohai datasets is fostering collaboration among people who work independently. Datasets are easy to use programmatically from the execution without the need to restructure your data." - Andres Hernandez, Principal Data Scientist, KONUX
Establishing ground truth, eliminating data inconsistency
The data in predictive maintenance is full of inconsistencies that are hard to escape.
For example, there might be a budget for doing one type of maintenance on the railway tracks 100 times a year and the other type of maintenance 50 times a year. If all the budget was used for the 100 maintenance rounds of the first type, the other 50 rounds of track maintenance of the second type would still be reported as done (because they need to be done anyway). Or, someone going to do the field maintenance at 3 am may forget to put in some data because they want to go home instead of filing in the documentation.
Cases like these create data inconsistency and, as a result, lead to the lack of ground truth.
And datasets become the crucial solution. Because they allow taking the human factor out of the loop to avoid mistakes.
Not so long ago, models at KONUX would produce clusters that had names not readable to humans. Thus, somebody had to check what the label assigned to the cluster was and if the cluster was clean or bad.
Right now, KONUX gets around 100 new measurements per day, meaning that about 100 trains are passing over the sensors. However, KONUX does not retrain models every day. The system does not shift or change so often.
This year, KONUX expects to increase the amount of data they collect by at least 10 times. This means it is no longer viable to have a human looking at the data. Thus, one needs to come up with the labels for models after training automatically.
To determine when the model needs to be retrained and whether it gets deployed automatically after quality assurance, data needs to be consistent. It is crucial that anyone is able to immediately go find what was the labeled dataset that has been used and what was the model in production. Because if something goes wrong, there is no time to investigate what happened through git. At that point, labeled datasets will become even more critical.
Summary
For KONUX, the Valohai datasets feature hit two birds with one stone:
Fostered collaboration among the team members through traceability, and
Helped to fight data inconsistency resulting from human errors by automating data collection and updates from the increased amount of sensors on railway tracks.
To learn more about Valohai Datasets, be sure to check out our dedicated page.
If you have any questions about the Valohai datasets feature or about the suitability of the platform for your use case, do not hesitate to book a call with our experts.