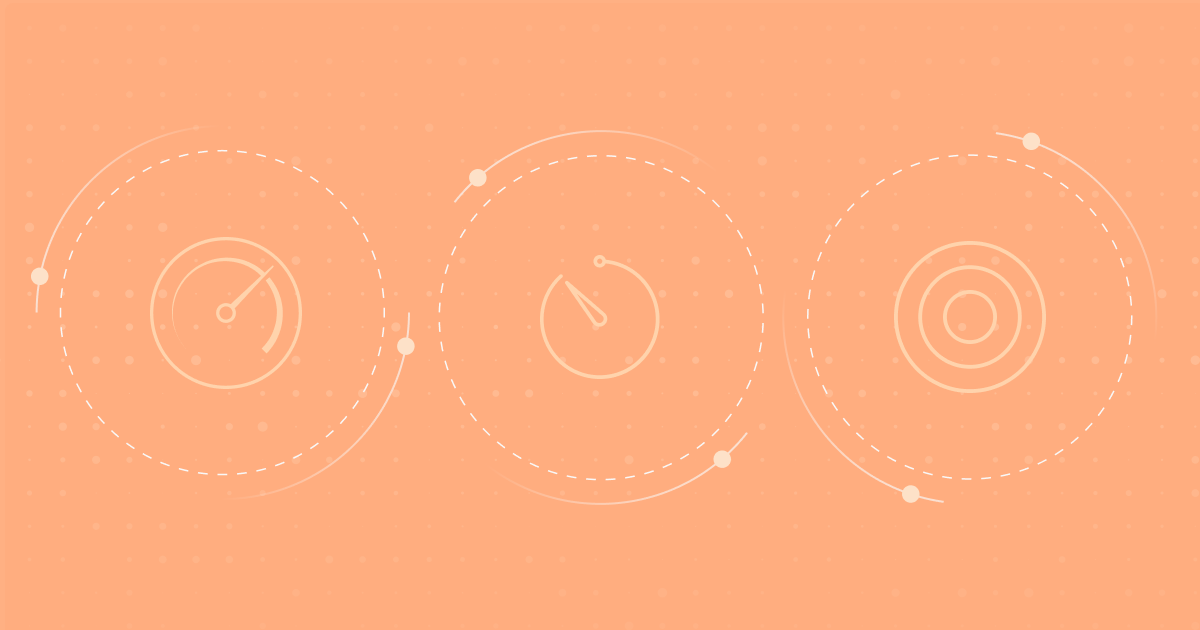
Business Value of MLOps
Viktoriya KuzinaIn 2020, Forbes estimated the market of MLOps solutions is expected to reach $4 billion by 2025. The recent VentureBeat article claims it will grow to over $6 billion by 2028. Let's look at what is the driving force for the demand of MLOps.
We have written a lot about all sorts of MLOps-related topics, from the basics of what MLOps is and what are its main principles to very specific cases like datasets for computer vision. Those were warmly welcomed by the tech people. But as a non-tech person, you might ask what's the business value of adopting MLOps solutions. Fair point!
How about we go this way: we'll explain why it makes sense to integrate MLOps practices into your business as well as provide you with specific cases and numbers that prove cost-saving and revenue growth in companies that have embraced MLOps. Then you can decide for yourself if it is worth a shot.
MLOps enables scale, scale drives business value
Let's cut straight to the case. A 2021 global study by McKinsey found that companies that successfully scale AI can add as much as 20% to their earnings before interest and taxes. However, scaling is not that simple. First you need to solve the siloing problem.
"Having different groups of people around the organization work on projects in isolation — and not across the entire process — dilutes the overall business case for ML and spreads precious resources too thinly." says Rokhit Mannikar et. al. for McKinsey.
If people and processes stay siloed, then improving the experimentation speed, testing and deployment times, and ensuring traceability and scalability, which are crucial points to get 20% earnings increase, is impossible. So, let's take a closer look at why that is the case.
1. Boosting the experimentation speed
For an ML pioneer, the rate of experiments equals the rate of success. You have to make many mistakes and break things before you figure out the optimal solution. Thus, one needs to be able to experiment fast and in parallel.
However, it puts pressure on tooling and infrastructure. A single laptop can do one experiment at a time and mostly sits idle, just waiting for someone to pour coffee on it. And what any business needs is a team of data scientists and minimum levels of manual technical busywork to allow massive parallelization in the cloud by having thousand of compute instances as readily available as a single one.
In other words, they need to be armed with a modern MLOps platform.
"An efficient machine learning team should be an experiment factory — conveyor belts and all. The faster we can create experiments, the better we are at our job." says Tapio Friberg, senior ML Engineer at ICEYE and a true ML Pioneer.
Take, for example, KONUX, leader in remote sensing that combines IIoT and machine learning to transform railway operations. Thanks to MLOps, their team can run 10 times more experiments with the same amount of effort.
2. Radically shortening testing and deployment times
Your models only bring value in production. Yet, it is easy to say "put your models into production", but actually putting models into production — not so much.
In May 2020, we surveyed data scientists, machine learning engineers, and managers about the major obstacles they are facing. Half of the respondents said that they were focused on developing models for production use, and over 40% said they would be deploying models for production.
Still, rumor has it that 60% of models never make it to production. Forbes cites even higher numbers of 78%. Can your business afford to take such risks?
MLOps allows organizations to improve the quality of models, speed up deployments, and reduce the risk of errors by automating the process from testing to monitoring. For Lifebit, getting on board with MLOps meant drastically shortening its model deployment times from two-three weeks to one day while sparing the need to hire more developers to maintain their infrastructure and deployments.
"We used to have an engineer or two allocated to support deploying models. With Valohai, anyone on the team can deploy independently. The reduction in overhead is immense." says Rishabh Shukla, the lead ML engineer at Lifebit.
3. Ensuring traceability and scalability
So, you have managed to speed up the experimentation rate, and you have models in production. Amazing! How do you keep tabs on all things big and small in all of your experiments and deployments from all the people across all the departments involved in the process?
You need some sort of knowledge repository to be able to provide transparency and traceability of the entire model development and deployment process. The knowledge base automatically builds up into the MLOps platform. So when people come or leave, you do not waste time on finding that one engineer who remembers what model was in production or spend weeks onboarding new people.
TL;DR
Let's review the actions and consequences chain.
Implementing MLOps solutions into your business means:
Quicker experimentation cycle -> More models
Quicker productization cycle -> More models in production
Full traceability for all models -> More models in production safely and scalably
That potentially boosts earning up to 20% as we have mentioned in the very beginning of this article. Moreover, you can more quickly and systematically solve business problems with AI.
Now what?
If you are not convinced by now about the value MLOps holds for your business, there is probably nothing we can do about it. Thank you for staying with us this far!
But if you are considering the implementation MLOps in your company, you might want to look at our guide for decision-makers on how to choose the right MLOps platforms. And if you think that building your own is better than buying, we cannot insist on anything. Let us leave just one last chart here:
Annual infrastructure costs for third-party machine learning operations (MLOps) solution versus building from scratch in organizations worldwide in 2020 (in million U.S. dollars)
"The annual infrastructure costs for third-party MLOps solutions are significantly less than the cost of companies building their own, according to respondents. The high estimate for buying a third-party solution is roughly 4.5 million U.S. dollars, while the high estimate for building a solution from scratch is 5.5 million U.S. dollars." (Statista)
We hope this was helpful. If this is all too much, you can always book a demo with one of our experts who can help you dissect your specific case and advice on what would be the right next steps to take.