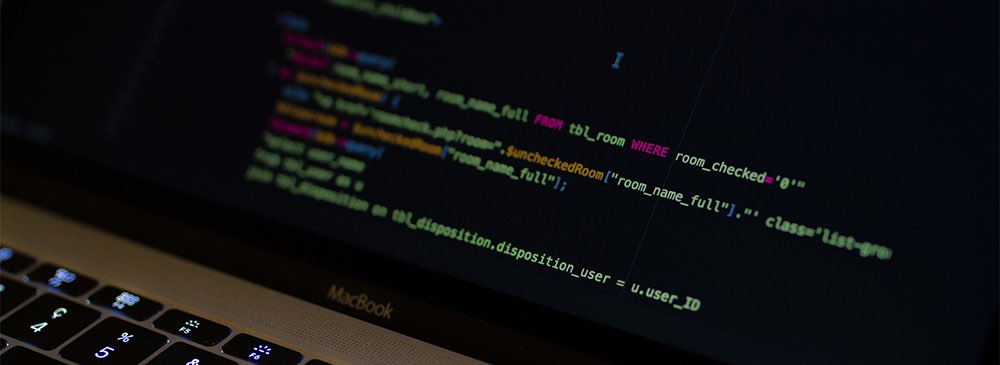
EU/US Copyright Law and Implications on ML Training Data
Vadym KublikWe may live in the era of “Big Data,” and yet the access to it is somewhat restricted; especially, when we talk about high-quality data. This blogpost will address the question of acquiring data for your Machine Learning projects from the perspective of EU and US copyright laws.
To begin with, data generated by humans and/or about humans normally have restrictions as to how it can be used. Moreover, while the internet is a global network giving you access to information from all over the world, laws governing that information are distinct to each country. A different jurisdiction can be only one click away.
In general, if it is personal information that can be used to specifically identify an individual, privacy and personal data protection rules apply. If it’s expressive material like images, literary works, music, etc. there are intellectual property (IP) rules to consider before making a copy. In Europe, the mere fact that information is aggregated in a database implies that it may be protected by so-called sui generis database rights. (Yes, you must respect other people’s investments.)
In some cases, it may be safer to train your model on synthetic data to avoid legal implications. While it may be a feasible solution regarding personal data concerns, the availability of synthetic expressive content is very limited. Indeed, there has been some progress recently with generating fake images , but it’s only a drop in the ocean of demand for expressive training data. Therefore, human-created content will still remain relevant for Machine Learning projects in the foreseeable future.
If you want to investigate synthetic datasets further, Valohai wrote a blog post about generating synthetic datasets with Unity.
Using copyright protected content in general
There are several important copyright-related points to keep in mind about creative content. First, copyright protection is inherently temporary, but the specifics vary from country to country and depend on the type of work. After copyright protection lapses, expressive work falls into the public domain, and anyone can freely use that work. Therefore, public domain content is safe to use in Machine Learning projects.
Second, many authors publish their works under the Creative Commons (CC) license. It helps creators to share their works with the general public while also enabling specifically control further use. Normally, permission must be obtained before copying a work - the CC license is a way for authors to make their work available under their own terms. For example, they can choose whether a work can be edited and/or used commercially. Therefore, CC-licensed materials can also be viewed as low-risk training data for AI, however, you should check some basic rules before using any specifically.
The third (and probably the most interesting point) is that under certain conditions, protected works still can be copied without the rightsholder’s permission. In Europe, it’s possible under limited exceptions for situations like quotation and parody.
Using copyright protected content for machine learning
Despite concerns about Machine Learning uses in the EU that have been growing for some time, it’s only been recently that member states have started adopting similar copyright exceptions. The UK first allowed unauthorized reproduction of copyrighted works for the purpose of non-commercial Text and Data Mining (TDM). France, Germany, and Estonia later followed suit. TDM is a general term covering various methods of computational analysis of information that include also Machine Learning and AI.
As European policymakers started to realize the importance of data access for AI development in the EU, they started proposing changes to EU copyright rules that would bind every Member State to adopt corresponding TDM exceptions. According to the latest text , the exception will allow everyone to mine content to which they already have access.
It’s important to note that rights holders will generally still have the right to restrict the usage of their works for mining purposes, just not in cases of use by non-profit research institutions. In other words, only research institutions will have the unlimited right to mine copyrighted content, while other actors still must respect the opt-out choice of the rightsholder. This limitation is meant to protect the interests of publishers that while charging subscribers for a “read access”, still want to reserve the right to charge them separately for the “right to mine”.
Before the upcoming TDM exception gets adopted EU-wide and implemented by every Member State, which is expected to happen no sooner than 2021, it’s still possible in some cases to rely on other copyright rules. In particular, a copyright exception allowing “temporary acts of reproduction” as prescribed by article 5(1) of the Information Society Directive .
Initially, this exception was called on to enable typical acts of internet browsing that presupposes the need to create temporary cached copies of webpages. Lesser known, however, is that this concept can also apply to copies made for the purpose of Machine Learning training data, provided they’re deleted as soon as the training process is completed. This deletion is an important step in the fulfillment of the exception’s precondition. More discussion on the applicability of this copyright rule can be found in the recent study Who owns AI ? .
Fair use doctrine in the US
Access to training copyrighted data does seem slightly more relaxed in the US. While their law doesn’t include any specific exceptions to cover Machine Learning, they instead enjoy a broad and flexible fair use doctrine that has proven favorable towards technological uses of copyrighted works. For example, copies made for image thumbnails, webpage caching, or creation of digital libraries are recognized as lawful under the fair use. The main idea is that the copy serves a different function from the original work and doesn’t create a substitution. (It is also known as “transformative use.”)
The question of whether the fair use doctrine should also apply to Machine Learning copies is still a subject of debate. However, in the light of the recent Google Books Case , the lawfulness of making copies to extract information seems clarified; it’s OK to copy a work to extract information not protected by copyright. It seems to cover Machine Learning uses also, where copyrighted works used as sources of data for pattern analysis aren’t explicitly covered by copyright rules.
By and large, scraping copyright-protected content from various internet sources to train your AI is not an outright infringement. But remember, different jurisdictions have different copyright policies, which are also far from being certain or uniform in this time of emerging AI technologies.